Accurate nitrogen monitoring and appropriate nitrogen management are crucial in California due to the state’s unique environmental challenges and agricultural practices. N is essential for crop growth, but excessive use can lead to serious environmental issues, including groundwater contamination and the emission of nitrous oxide, a potent greenhouse gas. The Central Valley, a major agricultural area, has seen significant N inputs from both synthetic fertilizers and manure, leading to widespread nitrate pollution in groundwater, a critical issue since many communities rely on groundwater for drinking. To address these challenges, California has enacted regulations that require more precise N applications aligned with crop needs. This initiative aims to reduce the amount of excess N that can leach into groundwater or run off into surface waters. The regulations, such as those detailed by the Central Valley Regional Water Quality Control Board, include requirements for growers to submit Nitrogen Management Plans that report both the N applied and the N removed by harvested crops. This approach helps calculate N use efficiency and identify areas where improvements are necessary.
Overfertilization poses several risks to both plants and the environment. In plants, excess N can cause stress and overproduction of leaves, making them more susceptible to diseases. It can also reduce yield and decrease quality, including organoleptic quality, and reduce the content of mineral nutrients and secondary metabolites. Additionally, high nitrate content in leaves can be harmful. Environmentally, excess N that remains unused in the soil can leach below the root zone or be lost through run-off, leading to nitrate accumulation in natural water bodies. This can cause algal blooms, eutrophication and acidification of freshwater lakes and coastal areas. Nitrate-contaminated drinking water requires expensive treatments, and nitrous oxide emissions from denitrification and manure decomposition processes on agricultural sites contribute to global warming. These emissions also negatively impact terrestrial and aquatic ecosystems.
Conversely, N deficiency negatively affects photosynthetic assimilation and reduces crop yield both in terms of quantity and quality. It restricts the development and growth of roots, suppresses lateral root initiation, increases the carbon-to-nitrogen ratio within the plant, reduces photosynthesis and results in early leaf senescence. Therefore, monitoring N levels accurately and managing them precisely is essential for maintaining the health of both crops and the environment, particularly in California, where agricultural practices must align with stringent environmental regulations.
Leaf Spectrometry
Leaf spectrometry is a powerful technique used to measure the spectral reflectance of plant leaves across a wide range of wavelengths. This process involves collecting hyperspectral data, which refers to the reflected light from leaves. Each leaf has a unique spectral signature that changes based on its biochemical and biophysical properties, such as leaf pigments, protein and carbon-based contents, and moisture content. By analyzing these spectral signatures, researchers can infer various properties of the leaves, which provides valuable insights into the plant’s health and nutritional status.
Several tools are commonly used to measure leaf spectral reflectance. One of the primary instruments is a spectrophotometer, which can capture detailed reflectance data across different wavelengths. Portable leaf spectrometers, used in many studies, offer a more convenient and flexible option for field measurements. These tools are designed to be lightweight and easy to use, making it possible to collect high-quality spectral data directly from the plants in their natural environment. By utilizing these advanced tools, researchers and growers can obtain precise information about leaf properties, enabling more accurate monitoring and management of plant health and nutrient levels.
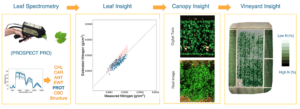
Practical Application and Implementation
Imaging spectroscopy and leaf spectrometry have vast potential applications in agriculture, particularly for N management in vineyards. These technologies allow growers to monitor vine health and nutrient status accurately, providing essential data that can lead to more precise and efficient decision-making and farming practices. Detailed spectral data reveals changes in leaf biochemical and biophysical properties, some of which are indicative of the vine’s N content.
Spectral modeling approaches for N retrieval have evolved significantly. Traditional methods often relied on simple correlations between basic vegetation indices or specific wavelengths and N levels. While these methods were quick, they could be overly simplistic. Modern approaches utilize advanced techniques, such as chemometrics and machine learning algorithms, to analyze spectral data. Chemometrics involves using statistical methods to extract meaningful information from complex spectral data, providing more accurate and comprehensive insights into N levels. Machine learning algorithms have become increasingly popular. These models can process large datasets and learn intricate patterns, offering high accuracy and robustness in N estimation.
Alternatively, physically based models, or radiative transfer models (RTMs), simulate the mechanism of light interaction with plant tissues to estimate biochemical/biophysical properties. These models are highly accurate and consistent and can be applied across various conditions, though they require detailed input data and calibration. Combining RTM with machine learning techniques, creating hybrid models, can further enhance their accuracy and applicability. Hybrid models use detailed simulations from RTMs to train machine learning algorithms, resulting in systems that can adapt to different environmental conditions and crop types. Implementing these advanced spectral sensing techniques in vineyards involves several practical steps. First, growers need to collect hyperspectral data from their crops using either portable ground-based spectrometers or aerial spectral cameras. This data is then analyzed using one of the above modeling approaches, whether it’s chemometrics, machine learning or a hybrid method. Depending on the modeling approach, the results can potentially provide detailed insights into the N status of the vines, allowing for precise and targeted fertilizer applications.
Case Study
We conducted a study to compare various analytical methods for N retrieval in grapevine leaves using hyperspectral data. Our primary goal was to determine the most effective approach for accurately and consistently estimating leaf N levels, considering both purely data-driven empirical methods and more sophisticated mechanistic models like PROSPECT and hybrid modeling techniques.
N in plants is primarily found in proteins, which are critical for various physiological processes. Proteins in the leaves contribute significantly to the N content, making the measurement of protein levels a consistent indicator of N status. Traditional methods often rely on the relationship between chlorophyll content and N, but this approach can be misleading due to the small proportion of N in chlorophyll and the dynamic nature of N allocation within the plant.
Empirical methods, such as those using simple vegetation indices like the Normalized Difference Vegetation Index (NDVI) and the Normalized Difference Red Edge (NDRE), have been widely used for estimating N content. However, these indices often fall short of providing accurate and consistent results for vine N content. NDVI and NDRE are sensitive to changes in chlorophyll content but do not capture the full complexity of N dynamics within the plant. Their reliance on a few spectral bands can lead to inaccuracies, particularly under varying environmental conditions and growth stages.
The PROSPECT model simulates how light interacts with leaf tissues, providing a more detailed and physically based approach to estimating N content. By incorporating specific absorption coefficients for proteins, PROSPECT can more accurately represent the biochemical processes within the leaves. This model showed robustness and transferability across different conditions but requires full band (400 to 2500 nm) and well-calibrated spectral data.
Hybrid modeling combines the strengths of both artificial intelligence and physically based approaches. By using detailed simulations from PROSPECT to train machine learning algorithms, hybrid models can achieve higher accuracy and adaptability. These models leverage the comprehensive understanding of light interactions provided by PROSPECT and the flexibility and learning capacity of machine learning techniques.
The advantages and disadvantages of four different spectral modeling approaches:
• Vegetation Indices (NDVI, NDRE, etc.): quick and easy to use but often inaccurate and inconsistent for detailed N estimation.
• Empirical Data-Driven Methods (Machine Learning): high accuracy with large datasets but can be prone to overfitting and may require extensive training data.
• Physically Based Models (PROSPECT): highly accurate and robust but complex and data-intensive.
• Hybrid Models: combines the best of both worlds, offering high accuracy and adaptability with a solid physical basis.
Our study demonstrated while traditional vegetation indices are useful for general assessments, they are insufficient for precise N management in vineyards. Advanced methods like RTM and hybrid models provide a more reliable and detailed understanding of N dynamics, paving the way for more effective and sustainable vineyard management practices. By leveraging these advanced analytical techniques, vineyard managers can optimize N use, improve crop health and reduce environmental impacts. If adopted by growers, this data-driven, decision-making vineyard management approach will support compliance with stringent environmental regulations, promote sustainability, drive the future of precision agriculture and optimize crop production.
Resources
The peer-reviewed paper: sciencedirect.com/science/article/pii/S0034425723005187#f0005
RTM interactive tool: digitalag.sf.ucdavis.edu/decision-support-tools/RTM
When2fly app: digitalag.sf.ucdavis.edu/decision-support-tools/when2fly